NextGen Precision Health Neuroscience Seminar – Sept. 18, 2023
NextGen Precision Health Neuroscience Seminar – Sept. 18, 2023
The goal of the NextGen Precision Health & Neuroscience Science Seminar is to highlight transdisciplinary precision research taking place in the field, provide opportunities for collaboration among researchers to build their own research efforts and promote clinical/researcher activity across the University of Missouri System and our partners.
For questions about this event, please reach out to Veronica Lemme at lemmev@health.missouri.edu.
“AI Foundation Models and Prompt-Based Learning for Analyses of Biomedical Data”
Presented by: Dong Xu, Ph.D., Curators’ Distinguished Professor, Paul K. & Dianne Shumaker Professor, Electrical Engineering and Computer Science, University of Missouri
Date: Sept. 18, 2023, 4-5 p.m.
Location: Tom and Linda Atkins Family Seminar Room, Roy Blunt NextGen Precision Health Building
Description
AI foundation models, trained on large-scale data, offer unprecedented opportunities for a range of applications. The potentials of these models are further magnified when combined with prompt-based learning, allowing for the achievement of state-of-the-art performance even with a small number of labeled data. This talk focuses on the biomedical applications of three foundation models: ChatGPT, Segment Anything Model (SAM) and Evolutionary Scale Modeling (ESM). We employed SAM to identify key attributes of pathway entities and their relationships from the figures of research papers. We queried ChatGPT to identify gene relationships by designing effective prompts. To optimize ChatGPT's responses, a novel iterative prompt refinement strategy was applied, in which the efficacy of these prompts was evaluated using metrics such as F1 score, precision and recall, and subsequently, the evaluation results were fed into ChatGPT to suggest better prompts. We also applied prompt-based learning for SAM-based protein identification from cryo-electron microscopy images. Furthermore, we prompted the ESM model for signal peptide predictions. The outcomes of our studies underscore the potential utilities of foundation models and prompt-based learning for efficient biomedical data analyses and predictions.
Speaker Bio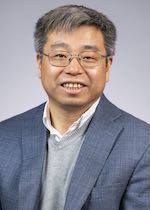
Dong Xu is a Curators’ Distinguished Professor in the Department of Electrical Engineering and Computer Science, with appointments in the Christopher S. Bond Life Sciences Center and the Informatics Institute at the University of Missouri. He obtained his Ph.D. from the University of Illinois, Urbana-Champaign in 1995 and did two years of postdoctoral work at the U.S. National Cancer Institute. He was a staff scientist at Oak Ridge National Laboratory until 2003 before joining the University of Missouri, where he served as department chair of computer science from 2007 to 2016 and director of the Information Technology Program from 2017 to 2020. Over the past 30-plus years, he has conducted research in many areas of computational biology and bioinformatics, including single-cell data analysis, protein structure prediction and modeling, protein post-translational modifications, protein localization prediction, computational systems biology, biological information systems, and bioinformatics applications in human, microbes and plants. His research since 2012 has focused on the interface between bioinformatics and deep learning. He has published more than 400 papers with more than 23,000 citations and an H-index of 80 according to Google Scholar. He was elected to the rank of American Association for the Advancement of Science Fellow in 2015 and the American Institute for Medical and Biological Engineering Fellow in 2020.